The Alchemy of Program Evaluation, Part 9: Triangulation and Mixed Methods
June 1, 2021 •Kassim Mbwana
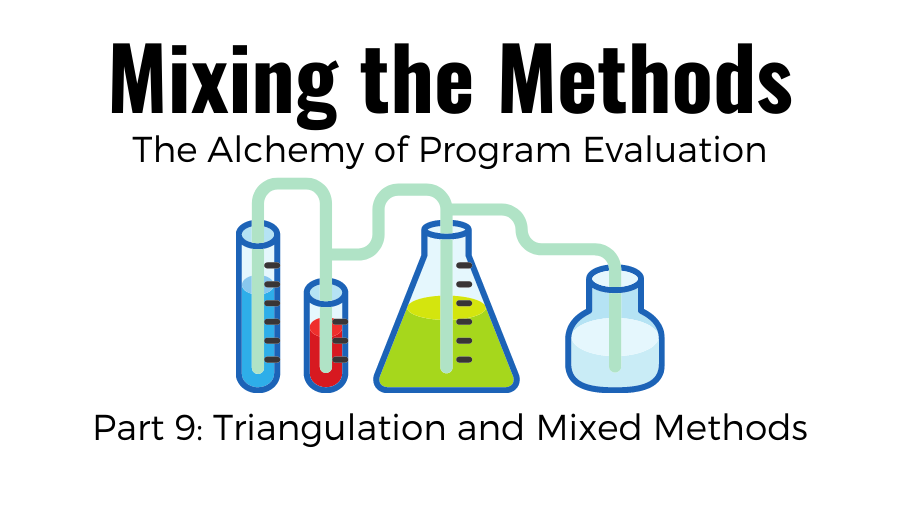
With contributions by Natalie Patten
Welcome to the final installment in our nine-part blog series on Summit’s program evaluation experience. We highlight Summit’s areas of program evaluation expertise beyond the core areas of data analytics and econometric analyses that the firm is known for. Ever wonder how Summit’s program evaluators can help solve your policy implementation and measure the outcomes? Then this blog series was for you. Here’s what we’ve covered thus far:
- Part 1 – Introduction
- Part 2 – Literature reviews and environmental scans
- Part 3 – Focus groups
- Part 4 – Semi-structured interviews
- Part 5 – Surveys
- Part 6 – Logic and theory of change models
- Part 7 – Administrative data
- Part 8 – Impact evaluations
In this final installment, we will bring it all together. How do we determine which methods are appropriate to answer specific research or policy questions, take all the information gathered through the various methods, and tell a story of what we observed? Let’s look at mixed-methods program evaluation and triangulation.
Mixed-Methods Program Evaluation
Mixed-methods program evaluation involves combining a variety of quantitative and qualitative evaluation approaches in a single evaluation to help us answer research questions and obtain a comprehensive understanding of the program’s processes, strengths, weaknesses, outcomes, and impacts. What are some of the reasons that would support combining the various program evaluation methods discussed in this series? Researchers such as J.W. Creswell and V.L. Plano Cark describe six potential scenarios in their 2011 volume on designing and conducting mixed-methods research:
- Because one data source may be insufficient to answer the research question. Examining administrative data to understand the differences across loan borrowers using the Paycheck Protection Program (PPP) may provide valuable insights on the gender distribution, types of businesses, or amount of funds borrowed. But what if we want to learn about why these groups were more likely not to use the PPP? Qualitative program evaluation methods, such as semi-structured interviews or focus groups with borrowers who decided not to use the program, would be valuable.
- To explain initial results or observations. Early observations of the PPP administrative data showed that large, well-established businesses seemingly had greater access to the program. Why were minority-owned businesses less likely to receive these loans? What were the factors driving this unexpected observation? Additional data collection, such as gaining insight into the loan application process via a logic model or focus groups with small-business applicants and those who did not apply, could shed some light.
- To generalize exploratory findings. Sometimes all the research questions are not known up front, and the researcher needs to engage in exploratory methods such as literature reviews and environmental scans or semi-structured interviews with key stakeholders to gain a more complete understanding of the factors or variables involved. Once the variables are established, they can be followed up with a survey administered to a larger population or analyzing available administrative data from an even larger population or data collection period.
- To enhance the study with a second method. Using a second method to complement earlier research could enhance understanding of the observed phenomenon. For example, to test the generalizability or reliability of benefits gained from being able to take advantage of PPP loans, researchers may engage in impact analyses using quasi-experimental approaches to determine whether the benefits to businesses would have been realized without the loan program—in other words, were there additional factors at play?
- To best test a theoretical stance. Sometimes there is an existing theory or working hypothesis driving the research questions that dictates the necessity to use both a quantitative and qualitative evaluation approach.
- To understand a research objective by undertaking the research in multiple phases, each using a different methodology. Some research questions require a multistep or phased approach, each with its own defining data collection and analysis method. Let’s say we wanted to understand the advantages and disadvantages of implementing a housing voucher program. The first phase of the project may be qualitative (stakeholder semi-structured interviews and logic models) to design the evaluation and data collection approach. A second phase might focus on implementing the distribution of the vouchers and measuring immediate outcomes (an outcome evaluation), while the third phase may be continuous data collection (a survey) on outcomes over a prolonged period. Finally, the project may conclude with secondary data analyses and cost-benefit analyses to explain findings and track benefits and challenges in the following years.
While these six criteria will help drive the decision on whether to engage in a mixed-methods evaluation approach, there are important steps to take to ensure successful execution of the project. Given the different types of questions each evaluation could answer and the depth of information that could be gathered, a well-thought-out evaluation begins with an evaluation design. Considerations such as sequencing to ensure one method informs the other is critical. In addition, it’s important to plan early on what types of analyses will be conducted to inform the research questions.
Triangulation
A critical component of the mixed-methods evaluation design is to establish validity of the quantitative and qualitative analytic mixed-methods approaches used. Validity in this context refers to how accurately the information gathered represents the participants’ perceptions of their experience and is credible to them. Triangulation is a validation procedure where researchers systematically search for convergence and corroboration among multiple and different sources of information to form themes or categories in a study—that is, multiple forms of evidence inform findings rather than a single data point.
For example, in a study to determine whether an agency’s career development and succession planning ensures that employees are appropriately hired, trained, and prepared to meet existing vacancies and growth opportunities, preliminary data gathering may begin with in-depth interviews with the Human Resources department to understand career paths at the agency. A review of documentation and developing a logic model of the growth opportunities would validate information shared by HR. Quantitative evaluation of administrative personnel data may explain characteristics of individuals being promoted, while an employee survey could address questions not answered by the administrative data. Finally, focus groups could be used to validate findings from prior evaluation approaches and close the loop on the evolution of the working hypothesis.
As you’ve learned, program evaluation is a valuable way to assess the effectiveness of policy and organizations, and evaluators have many different methods in their tool kit. In this series, we looked at seven different methods—literature reviews and environmental scans, focus groups, semi-structured interviews, surveys, logic and theory of change models, administrative data, and outcome and impact evaluations—that, when used together in a mixed-methods approach, can complement the core data analytics and econometrics practice that Summit is known for and ultimately make our overall program evaluation approaches more robust.
Get Updates
Featured Articles
Categories
- affordable housing (12)
- agile (3)
- budget (3)
- change management (1)
- climate resilience (5)
- cloud computing (2)
- company announcements (14)
- consumer protection (3)
- COVID-19 (7)
- data analytics (81)
- executive branch (4)
- fair lending (12)
- federal credit (29)
- federal register (2)
- financial institutions (1)
- Form 5500 (5)
- grants (1)
- healthcare (16)
- impact investing (12)
- infrastructure (13)
- LIBOR (4)
- litigation (8)
- machine learning (2)
- mechanical turk (3)
- mission-oriented finance (7)
- modeling (9)
- mortgage finance (10)
- office culture (26)
- opioid crisis (5)
- Opportunity Finance Network (4)
- opportunity zones (12)
- partnership (15)
- pay equity (5)
- predictive analytics (11)
- press coverage (2)
- program and business modernization (7)
- program evaluation (29)
- racial and social justice (8)
- real estate (2)
- risk management (10)
- rural communities (8)
- strength in numbers series (9)
- summer interns (7)
- taxes (7)
- white paper (15)