Impact Evaluations using Administrative Data: How the Synthetic Control Method Opens Doors to Otherwise Impossible Impact Evaluations
July 13, 2017 •Shane Thompson
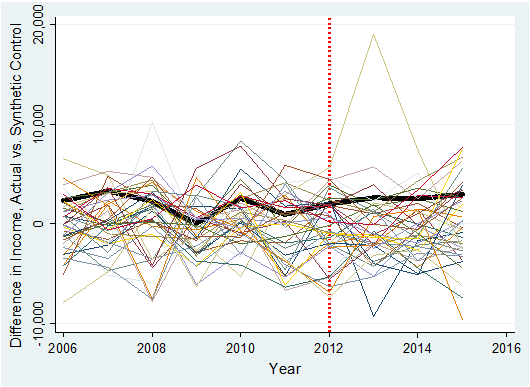
Summit's Dr. Shane Thompson writes about the importance of administrative data in impact evaluations in his three-part blog series. In his third blog, below, he writes about the synthetic control method. Those interested in reading more about program evaluation can download Summit's latest white paper here.
We noted in the last post that an impact evaluator’s challenge with administrative data is in identifying a control group. This post describes how an evaluator would use the synthetic control method to establish a control group and conduct an impact evaluation.
The synthetic control method is a relatively new methodology and few practitioners have it in their evaluation tool kit. The synthetic control method can address administrative data in ways that other, more established methods cannot.
Consider the following administrative data scenario that difference-in-difference, regression discontinuity, and propensity score matching could not evaluate effectively.
In this scenario, there are only 10 total treatment group observations over time, and the dataset is "small". None of the control groups, or even the average of all control groups, are similar to the treatment group in all relevant characteristics.
This dataset would be a lost cause without the synthetic control method, at least until more data were collected. The relatively few data elements and poor matching between treatment and potential control groups would invalidate any attempt at an impact evaluation. However, an evaluator equipped with the synthetic control method has a viable path forward.
I am going to describe how the synthetic control method works in practice on such a dataset by estimating the impact of Theo Epstein, the Chicago Cubs' general manager, on Illinois incomes.
First, some background. Theo Epstein was hired as the general manager of the Chicago Cubs in 2011. The Cubs were mired in a 103-year World Series drought at the time. Five years later in 2016, under Epstein’s management, the Cubs won the World Series. To Cubs fans, Epstein has supernatural powers.
But how far do these powers extend? I ask the following (admittedly ridiculous) question: Did Theo Epstein increase incomes in the state of Illinois?
To answer this question, I used state-level data from the American Community Survey from 2006 through 2015. My relevant variables are state-level income (dependent variable), age, education, marital status, and veteran status. The dataset is small (500 observations) and has only one treated unit (Illinois) with 10 total observations.
The treatment group is Illinois, the potential control groups are other states, the outcome of interest is state-level income, and the “treatment” is Theo Epstein.
The synthetic control method proceeds as follows:
- Construct a synthetic control Illinois, which is a weighted combination of several other states from the control pool, that is approximately equal to Illinois in pre-program income and characteristics.
- Construct placebo synthetic controls for all control states (they’re placebos because the control states were not treated with Theo Epstein.)
- Compare Illinois incomes to its synthetic control, and control states to their placebo synthetic controls. If the Illinois difference is more pronounced than what the control states experience, we attribute the difference to Theo Epstein.
First, let’s examine how well the synthetic control group for Illinois approximates Illinois in the pre-Epstein period.
The synthetic Illinois is nearly a perfect match to Illinois in the observable characteristics. Next, we check the income trends of Illinois and the synthetic Illinois.
We see that the income trends of Illinois and its synthetic control coincide well in the pre-Epstein period (to the left of the red dotted line). Taken together with the matched observable characteristics, we are confident that the synthetic Illinois is a good counterfactual for Illinois and plausibly represents what would have happened to Illinois incomes without the Epstein treatment.
Our next step is to construct placebo synthetic controls for all of the control states (states that did not have Epstein). After this, we examine the income gap between Illinois and the synthetic Illinois in the post-Epstein era (to the right of the red dotted line) and compare it to the income gaps that the control states experience with their respective placebo synthetic controls. If the income gap in Illinois is abnormally greater than the gaps in the control states, we attribute the Illinois gap to the treatment (Epstein).
The figure below presents the income gaps that exist in all states. Illinois is bolded in black.
We see that the income gap in Illinois in the post-Epstein era is not abnormal relative to other states that were not treated with Epstein (the placebo states). Because Illinois experienced income trends similar to non-treated states, we infer that Theo Epstein did not have an impact on state income.
Thus, contrary to popular belief among Cubs fans, Theo Epstein is not all-powerful. (Full disclosure: I am a White Sox fan).
The takeaway from this (admittedly trivial) example is that the synthetic control method makes impact evaluations possible on datasets for programs that are otherwise unevaluable.
Get Updates
Featured Articles
Categories
- affordable housing (12)
- agile (3)
- budget (3)
- change management (1)
- climate resilience (5)
- cloud computing (2)
- company announcements (14)
- consumer protection (3)
- COVID-19 (7)
- data analytics (81)
- executive branch (4)
- fair lending (12)
- federal credit (29)
- federal register (2)
- financial institutions (1)
- Form 5500 (5)
- grants (1)
- healthcare (16)
- impact investing (12)
- infrastructure (13)
- LIBOR (4)
- litigation (8)
- machine learning (2)
- mechanical turk (3)
- mission-oriented finance (7)
- modeling (9)
- mortgage finance (10)
- office culture (26)
- opioid crisis (5)
- Opportunity Finance Network (4)
- opportunity zones (12)
- partnership (15)
- pay equity (5)
- predictive analytics (11)
- press coverage (2)
- program and business modernization (7)
- program evaluation (29)
- racial and social justice (8)
- real estate (2)
- risk management (10)
- rural communities (8)
- strength in numbers series (9)
- summer interns (7)
- taxes (7)
- white paper (15)