Sampling Basics: What is Multistage Random Sampling?
January 15, 2015 •Tim Satterfield
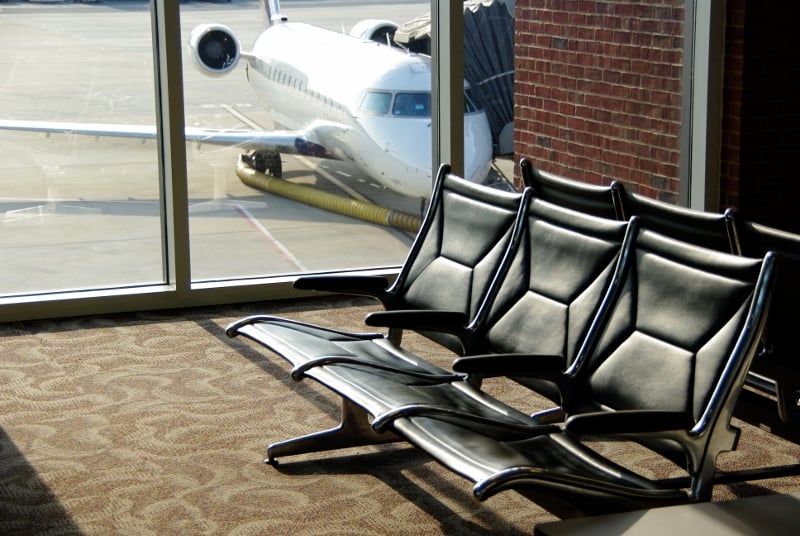
In the most recent sampling post, we discussed Stratified Random Sampling (StRS), which is a sampling design that can be used to ensure that specific subpopulations of interest are included in the sample. However, using either simple random sampling (SRS) or StRS may not resolve all issues for a sampling project. Using SRS or StRS may incur considerable costs, which exceed the project budget.
What is Multistage Random Sampling?
For example, consider a sample of 100,000 health claims, from 1,000 different hospitals, all of which are stored in file cabinets onsite. After sample selection, investigators will have to travel to every hospital that stores the sampled claims. Assuming a sample of 1,000 claims, using simple random sampling or stratified random sampling may result in 1,000 different hospital visits (potentially across many widespread locations) to investigate a single claim at each hospital, which would result in very high travel costs, evaluating one claim per visit. In the extreme, each sampled claim would require its own trip. Clearly, this is very inefficient. Multistage random sampling (MSRS) leverages the clustering of claims by hospital to reduce the number of trips necessary.
An MSRS design differentiates hospitals from claims. It first selects a fixed number of hospitals, or primary sampling units (PSUs). From each selected hospital, a sample of claims, or secondary sampling units (SSUs), are selected. By controlling the number of PSUs, which determines the number of trips necessary, a multistage sample could reduce the number of trips necessary for a given sample size.
Drawbacks to Multistage Random Sampling
Where is the catch? Using MSRS can generate estimates for a potentially lower cost than StRS and SRS, but the precision of estimates for MSRS samples are typically lower than StRS and SRS given the same number of samples. The loss of precision could be high if the number of PSUs is low, and the number of SSUs is high, (e.g. few hospitals but a large number of claims per hospital). The loss of precision is a function of relative similarity of SSUs within each PSU (i.e. intraclass correlation).
Multistage sampling designs are not limited to only two stages of selection. For especially complicated sampling designs, adding additional stages of selection may also save costs (for example, select metropolitan statistical areas, then select census tracts within the selected MSAs, and then select households within the sampled census tract). However, adding additional stages of sampling could increase total sampling error and lower the precision of the estimates. Under some circumstances, a carefully designed MSRS could keep the precision loss to a minimum.
How should you select a sample using MSRS?
Implementing an effective MSRS sampling design typically requires some knowledge about the different costs of evaluating the different sampling units, so as to maximize precision given the project's budget. This can be obtained from previous projects performed that are similar in scope. In addition, well-populated administrative data are necessary to establish PSU definitions for each SSU (for example, each claim will need to be associated with a specific hospital).
Determining the number of PSUs and SSUs within PSUs to select will differ depending on the costs of traveling to PSUs and reviewing the SSUs, as well as the underlying variability of the value of interest both across and within PSUs. For example, if the cost of travel is high, and the fraud rates are similar across hospital, selecting a small number of hospitals and a large number of claims per hospital may be appropriate. On the other hand, if the cost of travel is low, and different hospitals are expected to have a wide range of overall fraud rates, sampling a large number of hospitals and a relatively low number of claims per hospital may produce the most precise estimates.
This post was written with the help of Dr. Albert Lee.
Get Updates
Featured Articles
Categories
- affordable housing (12)
- agile (3)
- budget (3)
- change management (1)
- climate resilience (5)
- cloud computing (2)
- company announcements (14)
- consumer protection (3)
- COVID-19 (7)
- data analytics (81)
- executive branch (4)
- fair lending (12)
- federal credit (29)
- federal register (2)
- financial institutions (1)
- Form 5500 (5)
- grants (1)
- healthcare (16)
- impact investing (12)
- infrastructure (13)
- LIBOR (4)
- litigation (8)
- machine learning (2)
- mechanical turk (3)
- mission-oriented finance (7)
- modeling (9)
- mortgage finance (10)
- office culture (26)
- opioid crisis (5)
- Opportunity Finance Network (4)
- opportunity zones (12)
- partnership (15)
- pay equity (5)
- predictive analytics (11)
- press coverage (2)
- program and business modernization (7)
- program evaluation (29)
- racial and social justice (8)
- real estate (2)
- risk management (10)
- rural communities (8)
- strength in numbers series (9)
- summer interns (7)
- taxes (7)
- white paper (15)