Sampling Basics: What is Stratified Random Sampling?
December 16, 2014 •Tim Satterfield
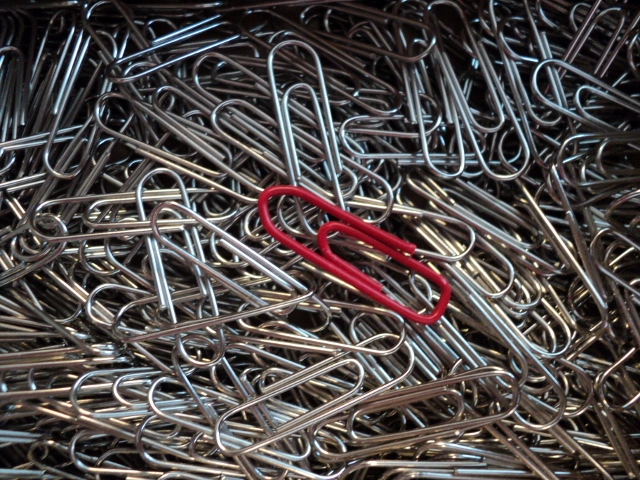
Stratified Random Sampling (StRS) is a type of random sampling where random samples are selected after first sub-dividing the population into groups, called strata. Like Simple Random Sampling (SRS), discussed in a previous post, all items in the population must have some chance of being selected. However, unlike SRS, the chance of each item being selected in an StRS does not need to equal the chance of any other item being selected. Instead, the probability of selection must only be equal within a strata (a single strata can also be called a “stratum”).
Why Use StRS?
There are two main reasons for StRS:
- The precision of the overall estimate is usually increased. The math behind StRS is fairly straightforward, but sufficiently technical that we won’t delve into it here. However, suffice it to say that if the sample size by strata is proportional to the population, the variance will be the same if the strata have nothing to do with the outcome being measured and lower if they have anything to do with the outcome being measured. In other words, it doesn’t hurt, and may help, to stratify.
- There may be a sub-population that needs to be estimated, but that sub-population is not likely to have a substantial number of items sampled if only an SRS is performed. Consider a situation where there is a sample of 100,000 health claims. While you may be interested in estimating the characteristics of the entire population, you are also interested in a subpopulation of MRI claims, of which there are 500. If a sample of 100 claims is selected using SRS, there would be zero MRI claims included in the sample about 61%* of the time. Even if a few of these claims were selected, it is unlikely that any meaningful estimates about MRI claims could be made. With StRS, the MRI claims can be placed into a strata and the sample size fixed at a level that would allow for precise estimation.
StRS Requirements
StRS has two requirements:
- The strata must be mutually exclusive (so no single item can be included in more than one group) and exhaustive (every item must be included in one of the groups) of the target population about which something is being estimated; and
- The sample within each strata is an SRS (i.e. a sample without replacement, with fixed sample size, and with fixed probability of selection).
How should you determine strata boundaries and sample sizes in StRS?
Using StRS effectively as a means to improve precision requires some knowledge about the population prior to sample selection. This is because the more similar items are within each strata, the more precise the overall estimate.
When data involve amounts (e.g. a population of possibly invalid healthcare claims), effective stratification can simply involve sub-dividing the population by amount paid. Textbooks such as William Cochran’s Sampling Techniques or Steven Thompson’s Sampling give relatively simple methods for determining strata boundaries and for determining appropriate sample size within each strata.
In more complicated cases, the groupings may be obvious but the sample size within each group may not be. Suppose we are sampling a population of healthcare claims for errors and we have both inpatient and outpatient claims. We have no idea what the error rate will be, but do believe it is likely to be different for inpatient claims than it is for outpatient claims even though the claims are all for similar total amounts.
In this example, we could logically stratify by whether the claim is an inpatient or outpatient claim, and simply pull a sample proportionate to population size in each strata. This stratified sample will fare no worse than simple random sampling and will be more precise if, in fact, the error rate differs between inpatient and outpatient claims.
*= (1-(500/99500))^100
This post was written with the help of Sejla Karalic and Dr. Alan Salzberg.
Get Updates
Featured Articles
Categories
- affordable housing (12)
- agile (3)
- budget (3)
- change management (1)
- climate resilience (5)
- cloud computing (2)
- company announcements (14)
- consumer protection (3)
- COVID-19 (7)
- data analytics (81)
- executive branch (4)
- fair lending (12)
- federal credit (29)
- federal register (2)
- financial institutions (1)
- Form 5500 (5)
- grants (1)
- healthcare (16)
- impact investing (12)
- infrastructure (13)
- LIBOR (4)
- litigation (8)
- machine learning (2)
- mechanical turk (3)
- mission-oriented finance (7)
- modeling (9)
- mortgage finance (10)
- office culture (26)
- opioid crisis (5)
- Opportunity Finance Network (4)
- opportunity zones (12)
- partnership (15)
- pay equity (5)
- predictive analytics (11)
- press coverage (2)
- program and business modernization (7)
- program evaluation (29)
- racial and social justice (8)
- real estate (2)
- risk management (10)
- rural communities (8)
- strength in numbers series (9)
- summer interns (7)
- taxes (7)
- white paper (15)