Sampling Basics: Myths about Statistical Sampling
January 26, 2015 •Balint Peto
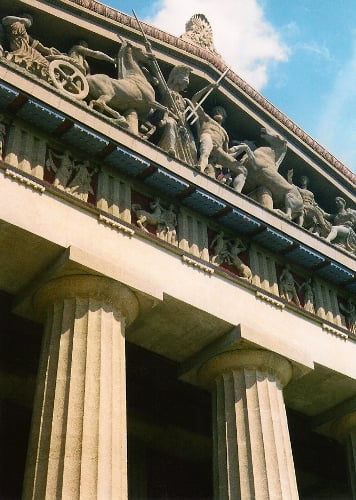
In Greek mythology, there is always some truth at the core of each story, or at least the reader can make some inferences about the way ancient Greeks thought about the world around them. In this post, we examine three sampling myths. And like with reading Greek mythology, we will attempt to reveal the thinking behind those myths. Our goal is to help overcome some of the misconceptions about statistical sampling and make the sample work for our research goals.
Myth 1: Double the population requires double the sample size
A common misconception about sampling is that when a population is doubled, the sample necessary to achieve the same precision goals should also be doubled.
The chart below depicts the required sample size of a simple random sample design for various population sizes. The sample sizes estimate a binary population parameter that is assumed to be 50%, with a confidence level of 95%, and a +/- 3% confidence interval that we are willing to accept in our estimates:
This chart tells us that in order to achieve the same precision in our estimates, the required sample size increases very slowly after the population threshold reaches about 40,000. In addition, the population can often be assumed to be infinite without a large impact to sample size requirements. For example, if our population increases from 15,000 to 20,000, the required sample size in the example only increases from 999 to 1,014. If the population was infinitely large, we could meet the precision goal with a sample of 1,068.
Note that in Summit’s sampling designs produced for clients, the margin of error surrounding the population estimate is not the only factor that determines sample size. For example, estimating the characteristics of multiple sub-populations with minimum precision goals may also increase sample size.
Myth 2: If the sample is large enough, non-response does not matter
It is also a popular myth that if we select a large enough sample, our estimates will not be affected by non-response. This is not true.
If, for example, survey respondents who are not college educated are more likely to decline response, our sample will underrepresent non-college educated individuals. This leads to biased estimates on the college education status of the U.S., no matter how many individuals we selected into our original sample, because now the true mean of college education status for our original sample differs from the respondent sample (people who actually responded). (Note that in our everyday practice at Summit, we use various methods to correct for non-response, such as non-response weights.)
Myth 3: Sampling misses important information
A popular myth about random samples is that they will miss phenomena that rarely occur, just because of their scarcity. For example, larger firms contribute X% of total firm income, but only account for Y% of total firms. If we want to estimate the total income of U.S. firms, and we choose a simple random sample of 1,000 firms, we may not capture any large firms in our sample.
However, there are sampling techniques to overcome this issue. If there are available data providing information that is believed to be correlated with the estimates of interest (in our example, perhaps number of employees), we can give firms with more employees a higher probability of being selected into the sample, and we should be able to include more ‘rare events’ in our sample. Another way to ensure sampling of large firms is to use stratified random sampling with employee size strata where we assign firms with specific employee sizes to an individual stratum. This way, we can make large firms more likely to be selected in the sample, so we’ll have to use sampling weights to correct for different probabilities of selection across strata.
As we have seen, contemporary methods can resolve many problems raised by sampling myths. Researchers simply need to adapt sampling techniques to achieve their research objectives.
The myths discussed here were taken from:
- Anton, Jon. Listening to the voice of the customer: 16 steps to a successful customer satisfaction measurement program. Purdue University Press, 1997.
- SAS Institute "Data Mining and the Case for Sampling: Solving Business Problems Using SAS® Enterprise Miner™ Software" Published 1998, Accessed December 2014. http://sceweb.uhcl.edu/boetticher/ML_DataMining/SAS-SEMMA.pdf.
This post was written with the help of Dr. Albert Lee.
Get Updates
Featured Articles
Categories
- affordable housing (12)
- agile (3)
- budget (3)
- change management (1)
- climate resilience (5)
- cloud computing (2)
- company announcements (14)
- consumer protection (3)
- COVID-19 (7)
- data analytics (81)
- executive branch (4)
- fair lending (12)
- federal credit (29)
- federal register (2)
- financial institutions (1)
- Form 5500 (5)
- grants (1)
- healthcare (16)
- impact investing (12)
- infrastructure (13)
- LIBOR (4)
- litigation (8)
- machine learning (2)
- mechanical turk (3)
- mission-oriented finance (7)
- modeling (9)
- mortgage finance (10)
- office culture (26)
- opioid crisis (5)
- Opportunity Finance Network (4)
- opportunity zones (12)
- partnership (15)
- pay equity (5)
- predictive analytics (11)
- press coverage (2)
- program and business modernization (7)
- program evaluation (29)
- racial and social justice (8)
- real estate (2)
- risk management (10)
- rural communities (8)
- strength in numbers series (9)
- summer interns (7)
- taxes (7)
- white paper (15)