Impact Evaluations Using Administrative Data: How Do I Transform Summary Statistics Into Actionable Evidence?
June 27, 2017 •Shane Thompson
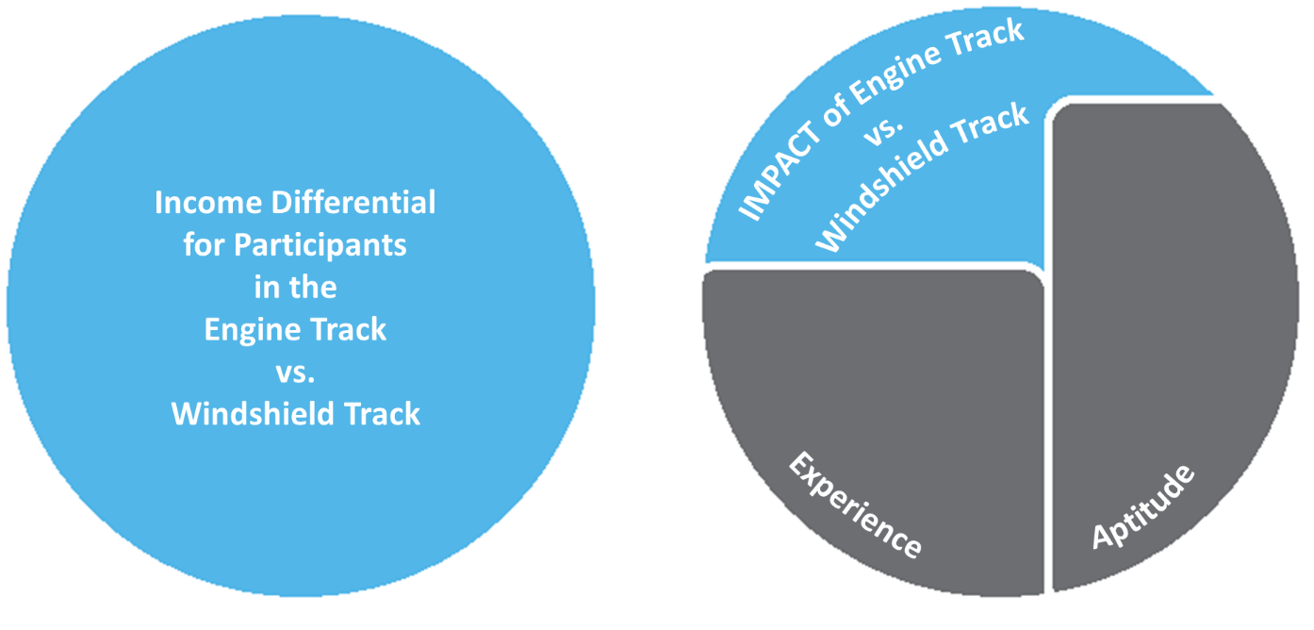
Summit's Dr. Shane Thompson writes about the importance of administrative data in impact evaluations in his three-part blog series. In his first blog, below, he writes about transforming summary statistics into actionable evidence. Those interested in reading more about program evaluation can download Summit's latest white paper here.
Let’s say you’re buying a used car. With a superficial inspection, you know whether the car is clean or messy, big or small, old or new, etc. You can lift up the hood and assess (or in my case, pretend to assess) the mechanical components to learn even more. But you’ll have no idea how it drives (or even if it will start!) until you turn the key.
Policy makers and program officers often stand in front of a “used car” of administrative data. Sometimes the program execution and data collection have yielded a beat-up El Camino. Other times they produce a souped-up Ferrari. In either case, superficial inspections (i.e., summary statistics) of the administrative program data may only tell part of the story and could misrepresent the facts. Summary statistics do not provide actionable evidence.
Impact evaluations are the keys that policy makers and program officers turn to obtain actionable evidence.
Thanks to an array of innovative econometric and statistical methodologies, impact evaluations are often feasible on both the El Caminos and Ferraris of administrative data. We’ll cover some of these methodologies in the next post of this blog series. For now, let’s focus on the pitfalls of substituting summary statistics for impact evaluations.
Let’s say we have administrative data on a job training program, and to keep with the car theme, let’s assume all participants are aspiring car mechanics. Participants can choose between two training tracks: engine repair and windshield repair. Five years after the program, we measure participant incomes and calculate the difference in income between participants in the two tracks. If a differential exists, can we attribute it to the type of training received?
The answer is no. The differential is merely a summary statistic of the two training types, not the impact of one relative to the other. The reason is that participants are not randomly assigned into a training track. They select tracks according to their preference.
To correctly disentangle the impact of the training itself, we need to net out the pre-training differences between engine track participants and windshield track participants. For the sake of this example, let’s assume that the technical aspects of the engine repair track will likely appeal to more skilled mechanics with greater overall aptitude. Let’s say it also draws in mechanics who have more experience than those who attend the windshield track.
Accordingly, regardless of the training received we would expect the participants in the engine repair track (with higher aptitude and more experience) to have higher incomes five years from now than those who participated in the windshield repair track.
The figure below demonstrates how the misuse of summary statistics may falsely attribute impacts to programs. The left circle represents the income differential between engine track participants and windshield track participants. The right circle shows the impact on income of participating in the engine track instead of the windshield track, which is the income differential that exists after netting out the effects of experience and aptitude.
When nothing is on the line, it may be sufficient to just kick the tires and make sure the radio works in your administrative data car. But if you’re seriously considering a purchase, if you really need to measure quality, you have to know how well the car runs. You’ve got to turn the key.
Interested in reading more about this topic? Dr. Thompson co-authored a white paper on estimation designs used in program evaluation, which can be downloaded below.
Get Updates
Featured Articles
Categories
- affordable housing (12)
- agile (3)
- budget (3)
- change management (1)
- climate resilience (5)
- cloud computing (2)
- company announcements (14)
- consumer protection (3)
- COVID-19 (7)
- data analytics (81)
- executive branch (4)
- fair lending (12)
- federal credit (29)
- federal register (2)
- financial institutions (1)
- Form 5500 (5)
- grants (1)
- healthcare (16)
- impact investing (12)
- infrastructure (13)
- LIBOR (4)
- litigation (8)
- machine learning (2)
- mechanical turk (3)
- mission-oriented finance (7)
- modeling (9)
- mortgage finance (10)
- office culture (26)
- opioid crisis (5)
- Opportunity Finance Network (4)
- opportunity zones (12)
- partnership (15)
- pay equity (5)
- predictive analytics (11)
- press coverage (2)
- program and business modernization (7)
- program evaluation (29)
- racial and social justice (8)
- real estate (2)
- risk management (10)
- rural communities (8)
- strength in numbers series (9)
- summer interns (7)
- taxes (7)
- white paper (15)