How to Use Administrative Data for Enforcement Analytics
October 1, 2015 •Albert Lee
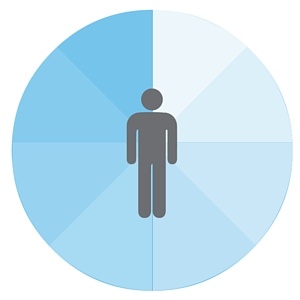
Federal agencies collect vast amounts of data as part of their administrative duties. ("What is Administrative Data?") The U.S. Department of Labor requires retirement plans of a certain size to file an annual report (Form 5500) describing changes in assets and number of participants. The U.S. Security and Exchange Commission (SEC) examines publicly traded companies using Form 10-K, which summarizes companies’ financial performance. The U.S. Internal Revenue Service (IRS) is well known for the myriad of forms it uses to collect details about filing subjects.
Each year, enforcement agencies conduct investigations into potential wrongdoing. These investigations, along with random audits, result in enforcement actions. Agencies collect data describing these enforcement outcomes that define the infraction and its severity and detail any civil monetary penalties. These outcomes also become part of the administrative dataset.
Common Attributes of Administrative Data
Regardless of which agency collects the information, administrative data share a few common features:
Administrative data are plentiful. Many agencies have been collecting data for decades and require frequent and mandatory filings.
- Administrative data are messy. Data quality varies from form to form, year to year, and subject to subject.
- Duplicates and omissions are common in administrative data. Partial filings and amendments cause duplicates, and some subjects fail to file despite the requirements.
- Laws, rules, and regulations (and their interpretations) about administrative data have evolved over time. Without careful preparation, administrative data are—at best—a snapshot of a subject’s record in a single point in time.
Combining and Integrating Administrative Data
With careful preparation, agencies can combine their administrative data into tremendously valuable databases. Filings from the same subjects across years can create a longitudinal panel, which captures the changing profile of a filing subject across time. Agencies can collate enforcement outcome data horizontally with the filings from many different people or companies. This type of horizontal analysis can determine whether certain filing characteristics that trigger an investigation correlate with actual infractions. By combining vertical and horizontal views of data, researchers can identify important statistical patterns about the enforcement subjects in general and infractions in particular.
Identifying Inconsistent Data
Moving from data preparation to a successful data integration is a multi-step endeavor. Although many factors determine success, my experience has shown that consistency of data elements is key. Here are a few situations that can lead to inconsistency:
- Filing subject identifiers can change across time through mergers and other changes in ownership control.
- Entities with long filing histories can appear as new entrants, which can lead to misaligned filing history.
- Filing requirements can change over time. Some previously required variables are no longer required, and new requirements arise. This inconsistency can lead to discontinuity in the availability of information.
- Changes to laws, rules, and regulations can alter the definition of an infraction. What an agency once considered compliant is now an infraction; last year’s infractions become this year’s compliance. These changes can lead to an inconsistent interpretation of available data.
In conclusion, integrating administrative data is the useful first step leading to the Predictive Analytics Cycle (PAC). Agencies can integrate relevant data longitudinally by stacking together chronological data from filing subjects, and they can integrate horizontal data by merging relevant enforcement outcomes to the appropriate filing data. By remaining mindful to the inconsistencies that prevent successful data integration, agencies can gain insights while optimizing scarce resources.
To learn more, see our past posts about predictive analytics.
Subscribe to the Summit blog above to receive future posts in this series.
Get Updates
Featured Articles
Categories
- affordable housing (12)
- agile (3)
- budget (3)
- change management (1)
- climate resilience (5)
- cloud computing (2)
- company announcements (14)
- consumer protection (3)
- COVID-19 (7)
- data analytics (81)
- executive branch (4)
- fair lending (12)
- federal credit (29)
- federal register (2)
- financial institutions (1)
- Form 5500 (5)
- grants (1)
- healthcare (16)
- impact investing (12)
- infrastructure (13)
- LIBOR (4)
- litigation (8)
- machine learning (2)
- mechanical turk (3)
- mission-oriented finance (7)
- modeling (9)
- mortgage finance (10)
- office culture (26)
- opioid crisis (5)
- Opportunity Finance Network (4)
- opportunity zones (12)
- partnership (15)
- pay equity (5)
- predictive analytics (11)
- press coverage (2)
- program and business modernization (7)
- program evaluation (29)
- racial and social justice (8)
- real estate (2)
- risk management (10)
- rural communities (8)
- strength in numbers series (9)
- summer interns (7)
- taxes (7)
- white paper (15)