Three must-haves in pay equity analyses
November 5, 2020 •Corey West
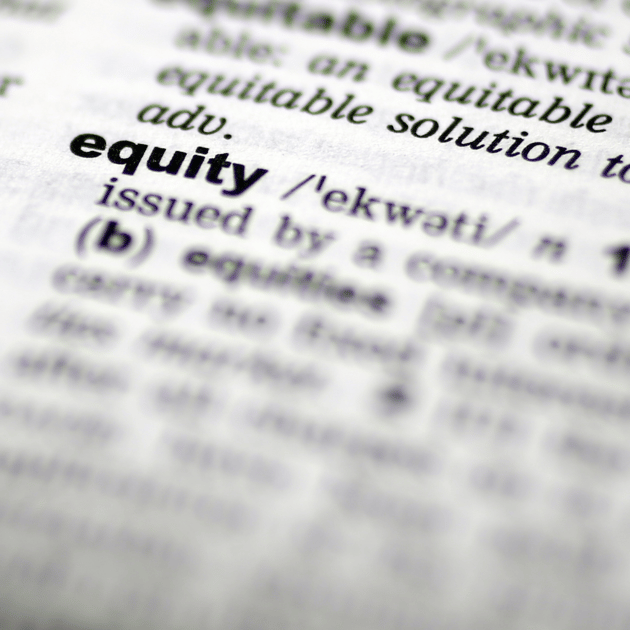
In this two part pay equity blog series Summit Consulting Senior Manager Corey West (who leads Summit's internal pay equity analyses) and Salt Hill's Alan Salzberg discuss the must haves for conducting a comprehensive and reliable pay equity study. The second release in the series will be a brief case study highlighting the implementation of the must haves.
Pay equity is good business because companies with pay equity protocols attract and retain top talent. To be successful in this area, companies should proactively assess their performance on a regularly basis using quantitative and qualitative data.
In this blog post, we describe three must-haves for comprehensive, cost-effective pay equity assessments: descriptive employee data, an appropriate statistical model, and subgroup analyses.
Companies that apply these must-have elements will have robust statistical evidence of pay equity (or inequity). If the organization detects pays inequities, they can readily identify and remedy the disparity in a focused and efficient manner (i.e., without a complete salary restructuring).
Must-Have #1: Descriptive Employee Data
Pay equity analyses must consider all factors that determine employee salary. Simple comparisons of salary differences between male/female, black/white, older/younger employees are insufficient. This is because employee salaries are determined by multiple factors. Failing to account for all these factors can lead to unreliable conclusions.
So, what data are necessary? At a minimum, we need data on employee demographics, experience, education, time at the company, and job type.[1] These components mitigate false attributions and are essential in providing statistical evidence of pay equity.
Note: No dataset is perfect, and what we have available in payroll data may not tell the whole story. There will almost always be unobservable or difficult-to-quantify characteristics (e.g., teamwork, attitude, or leadership ability). Often, we can still incorporate such qualitative factors into the analysis using advanced methods, even if they’re measured imperfectly.
Must-Have #2: An Appropriate Statistical Model
Pay equity analyses must use statistical models to isolate the drivers of pay (in)equity. With descriptive employee data at our disposal and an appropriate statistical model, we can separate the influence of gender, race, or age on salary while simultaneously accounting for other potential contributors.
Suppose, for example, that in our company data we observe higher average salaries for male employees. Is this, on its own, evidence of wage discrimination? Not necessarily, because our analysis has not yet accounted for other differences that might exist between male and female employees.
As we examine the (hypothetical) data further, we may uncover that male employees are, on average, more experienced and educated than their female counterparts at the company. Suppose that:
- Male employees have more average experience than female employees.
- More male employees work at a branch of the company in an expensive metropolitan area.[2]
- A higher proportion of male employees have master’s degrees than female employees.
- A higher proportion of male employees work in high-tech positions than female employees.[3]
If this is the case, we would expect male employees to have higher salaries than female employees based on qualifications, not solely due to gender. The figure below highlights how statistical models separate a true effect of gender from other effects.
The first large, blue circle represents the raw difference in the salary between male and female salaries before accounting for other factors. As we add factors to our model, we see that the original differential, which we may have naively attributed solely to gender, is also driven by experience, location, education, and occupation. Once we account for these additional factors, the amount of the differential directly attributable to gender has diminished substantially. Because we have an appropriate statistical model, we can determine whether the diminished effect of gender is statistically different from zero, (i.e., whether there is indeed statistical evidence of gender pay inequity after accounting for all other factors).
In summary, a comprehensive statistical model produces two things raw data are unable to produce: one, an estimate of gender pay inequity that simultaneously accounts for other relevant factors; and two, a determination whether that estimate is statistically significantly different from zero.
Note: In the hypothetical example above, gender pay inequity was non-existent after removing the influence of other factors. However, in some cases when gender pay inequity is present, accounting for the influence of other factors may actually INCREASE the effect of gender from its raw starting point (the first blue dot). Or a naïve model showing no inequity could actually reveal statistically significant pay equity once accounting for education, experience, location, and other factors. Statistical models have no innate bias in any one direction.
Must-Have #3: Subgroup Analyses
Pay equity analyses must also examine subgroups for pay inequity. Universal, company-wide analyses are necessary but insufficient. It could be that the overall company exhibits no gender pay inequity but that certain subgroups of employees do. Conversely, companies may indeed have gender pay inequity at the firm level but find it to be entirely driven by a certain subgroup of employees.
In either case, we must design our model to ensure that we can ultimately identify the drivers of pay inequity: Is pay inequity confined to a specific job type? Is pay inequity only found among longer-tenured employees?
When the model includes relevant subgroups, companies can make targeted, cost-effective compensation adjustments to remedy pay inequities wherever they may exist.
Note: As we examine subgroups, we should incorporate sensitivity analyses and statistical matching. Statistical testing cut-offs need to appropriately account for the fact that multiple groups are being tested. Sensitivity analysis can answer questions that the primary statistical model cannot: Do our results change as we make small adjustments to the model or the subgroups we study? Are there pay differences between a small group of cohorts that warrants comprehensive reviews of employment folders and interviews of colleagues to gather qualitative data? Ultimately, the goal is to obtain results that have been vetted by multiple approaches for assessing the organization’s pay equity performance.
Conclusion
Statistical evidence of pay equity can ensure companies are appropriately valuing the contributions of each member of their staff. This practice increases the likelihood of hiring and retaining the labor market’s top candidates. In the absence of these must haves (descriptive employee data, an appropriate statistical model, and subgroup analyses), pay equity analyses are incomplete—and worse, inconclusive.
[1] An Equal Employment Opportunity Commission (EEOC) fact sheet notes that “[i]t is job content, not job titles, that determines whether jobs are substantially equal.” Accordingly, we must often break down job type by skills required, effort exerted, level of responsibility, working conditions, and establishment type. See https://www1.eeoc.gov//eeoc/publications/fs-epa.cfm?renderforprint=1.
[2] Job location could be subject to discriminatory practices.
[3] Job placement could be subject to discriminatory practices.
Get Updates
Featured Articles
Categories
- affordable housing (12)
- budget (1)
- climate resilience (5)
- cloud computing (2)
- company announcements (13)
- consumer protection (3)
- COVID-19 (7)
- data analytics (81)
- executive branch (4)
- fair lending (12)
- federal credit (25)
- federal register (2)
- Form 5500 (5)
- grants (1)
- healthcare (16)
- impact investing (12)
- infrastructure (12)
- LIBOR (4)
- litigation (8)
- machine learning (2)
- mechanical turk (3)
- mission-oriented finance (6)
- modeling (7)
- mortgage finance (9)
- office culture (25)
- opioid crisis (5)
- Opportunity Finance Network (4)
- opportunity zones (12)
- partnership (15)
- pay equity (5)
- predictive analytics (11)
- program and business modernization (3)
- program evaluation (26)
- racial and social justice (8)
- real estate (2)
- risk management (8)
- rural communities (7)
- strength in numbers series (9)
- summer interns (7)
- taxes (6)
- white paper (13)